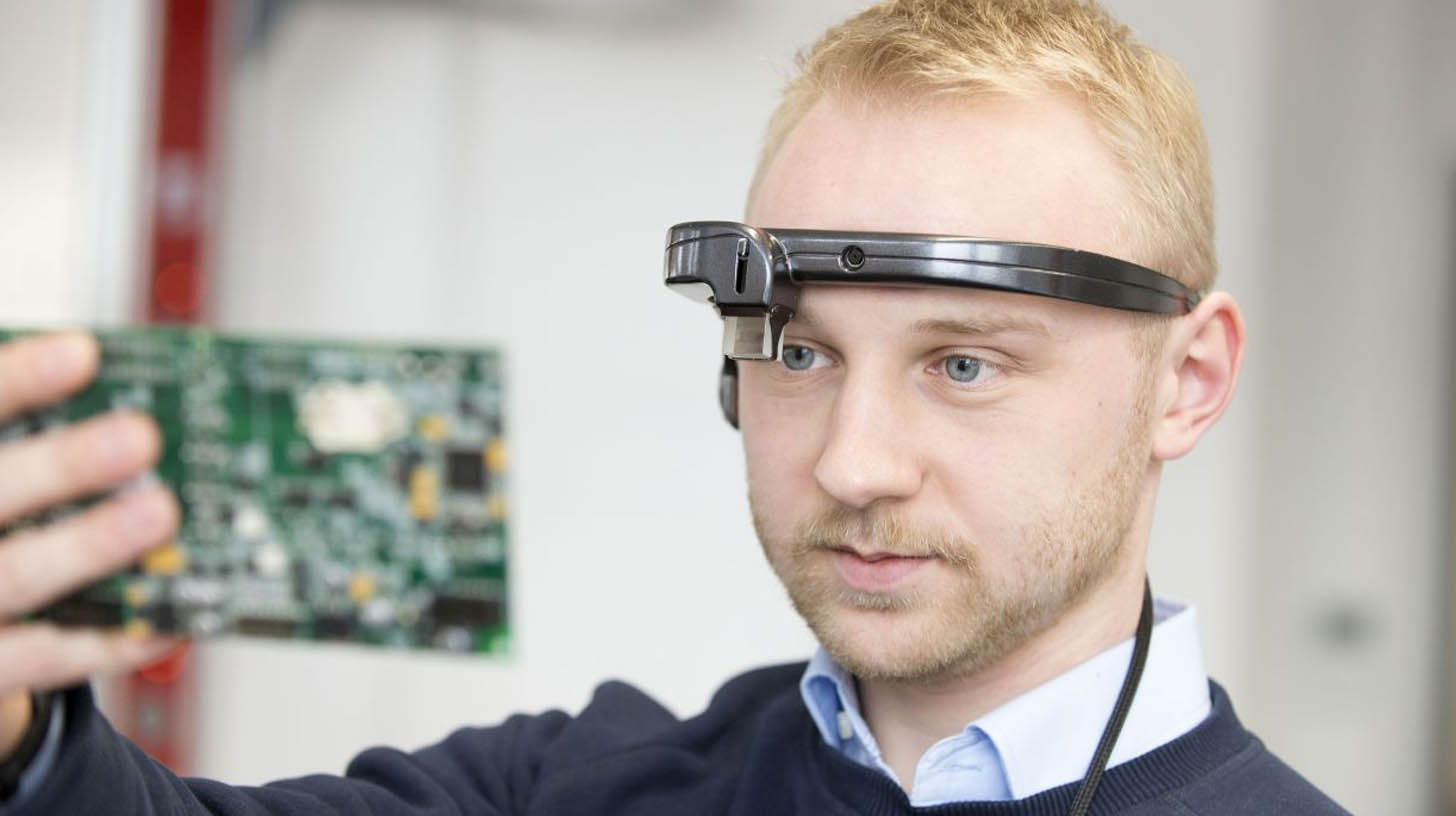
How Trustworthy Are Large Language Models Like GPT
More people feel comfortable outsourcing important projects to AI; new research shows why we shouldn’t.
Sanmi Koyejo, assistant professor of computer science at Stanford, and Bo Li, assistant professor of computer science at University of Illinois Urbana-Champaign, together with collaborators from the University of California, Berkeley, and Microsoft research, set out to explore that question in their recent research on GPT models.
“Everyone seems to think LLMs are perfect and capable, compared with other models. That’s very dangerous, especially if people deploy these models in critical domains. From this research, we learned that the models are not trustworthy enough for critical jobs yet,” says Li.
Read the full study: DecodingTrust: A Comprehensive Assessment of Trustworthiness in GPT Models
Focusing specifically on GPT-3.5 and GPT-4, Koyejo and Li evaluated these models on eight different trust perspectives – toxicity, stereotype bias, adversarial robustness, out-of-distribution robustness, robustness on adversarial demonstrations, privacy, machine ethics, and fairness – asserting that, while these newer models achieve reduced toxicity compared with prior models on standard benchmarks, they can still be easily misled to generate toxic and biased outputs, and to leak private information from training data and user conversations. Their study was published on preprint service arXiv.
“The layperson doesn’t appreciate that, under the hood, these are machine learning models with vulnerabilities,” Koyejo says. “Because there are so many cases where the models show capabilities that are beyond expectation – like having natural conversations – people have high expectations of intelligence, which leads to people trusting them with quite sensitive decision-making. It’s just not there yet.”